9 Descriptive Statistics: Categorical
For this section we use the Titanic data set. The lines below imports into R the data and converts some of the variables to factors.
titanic2 <-
haven::read_dta("./Data/titanic2.dta") %>%
mutate(sex = haven::as_factor(sex),
died = haven::as_factor(died),
age = haven::as_factor(age),
class = haven::as_factor(class)) %>%
haven::zap_labels()
We begin by visualizing the first 6 rows of the data
titanic2 %>% head()
class | age | sex | died |
---|---|---|---|
first | adult | male | No |
first | adult | male | No |
first | adult | male | No |
first | adult | male | No |
first | adult | male | No |
first | adult | male | No |
And then summarize the entire data
titanic2 %>% summary()
class age sex died
first :325 child: 109 female: 470 No : 711
second:285 adult:2092 male :1731 Yes:1490
third :706
crew :885
9.1 Single Categorical Variable
9.1.1 Frequencies & Proportions
The most common modality for presenting a single categorical variables is tabulating the observations, and subsequently expressing these frequencies as proportions or percentages. This is done below
titanic2 %>%
gtsummary::tbl_summary(
include = class,
digits = class ~ c(0,1)
) %>%
gtsummary::bold_labels()
Characteristic | N = 2,2011 |
---|---|
Passenger's cabin class | |
first | 325 (14.8%) |
second | 285 (12.9%) |
third | 706 (32.1%) |
crew | 885 (40.2%) |
1 n (%) |
9.1.2 Graph - Barchart
We first summarize the data
bar_data <-
titanic2 %>%
drop_na(class) %>%
count(class) %>%
mutate(perc = `n` / sum(`n`)) %>%
arrange(perc) %>%
mutate(labels = paste(n, " (", scales::percent(perc), ")", sep=""))
bar_data
class | n | perc | labels |
---|---|---|---|
second | 285 | 0.129 | 285 (12.9%) |
first | 325 | 0.148 | 325 (14.8%) |
third | 706 | 0.321 | 706 (32.1%) |
crew | 885 | 0.402 | 885 (40.2%) |
And the plot the barplot
bar_data %>%
ggplot() +
geom_bar(stat = "identity",
aes(y = n, x = class, fill = class),
col = "black",
show.legend = F) +
geom_label(aes(y = n, label = labels, x = class),
vjust = 1.2,
show.legend = FALSE, size=3.5) +
labs(x = NULL,
y = "Count",
title = "Distribution of Class of passenger") +
theme_bw()
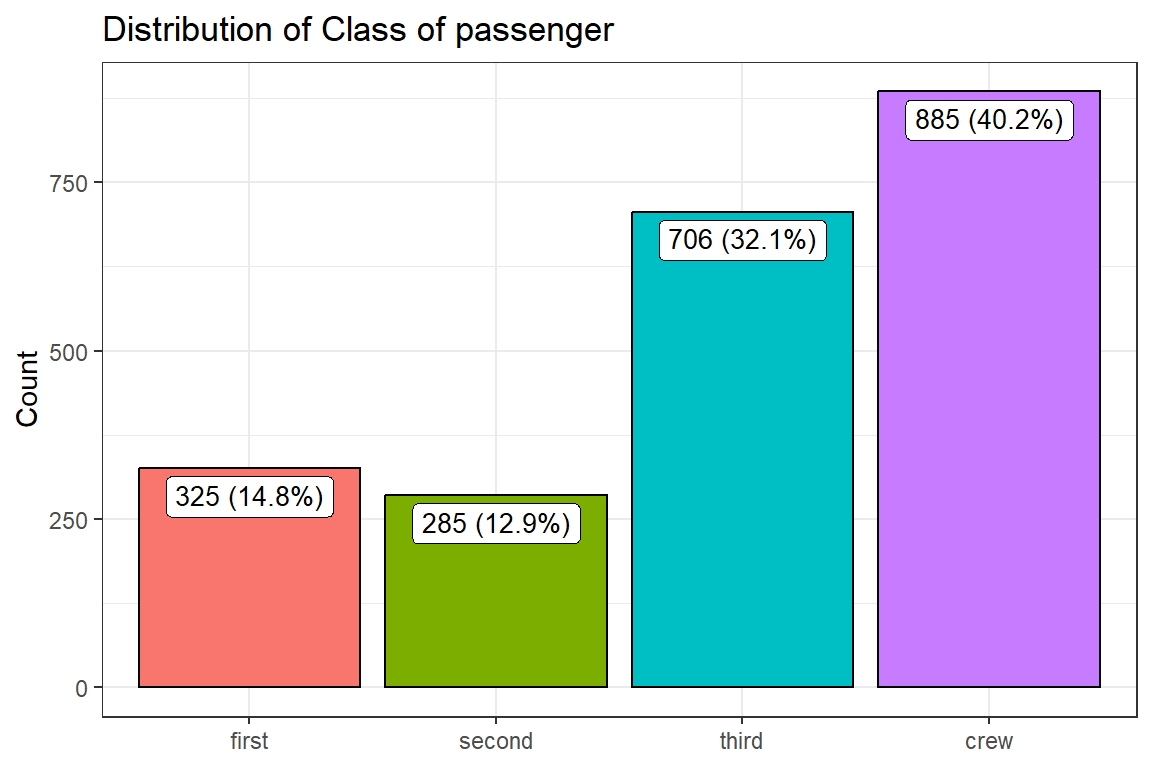
9.1.2.1 Pie Chart
To do this we use the previously summarized data. Then we draw a customised Pie Chart
bar_data %>%
ggplot(aes(x = "", y = perc, fill = class)) +
geom_col() +
geom_label(aes(label = labels),
position = position_stack(vjust = 0.5),
show.legend = FALSE, size =3) +
coord_polar(theta = "y", start=0) +
labs(title = "Distribution of Blood Groups of study participants",
fill = "Blood Group") +
theme_void()
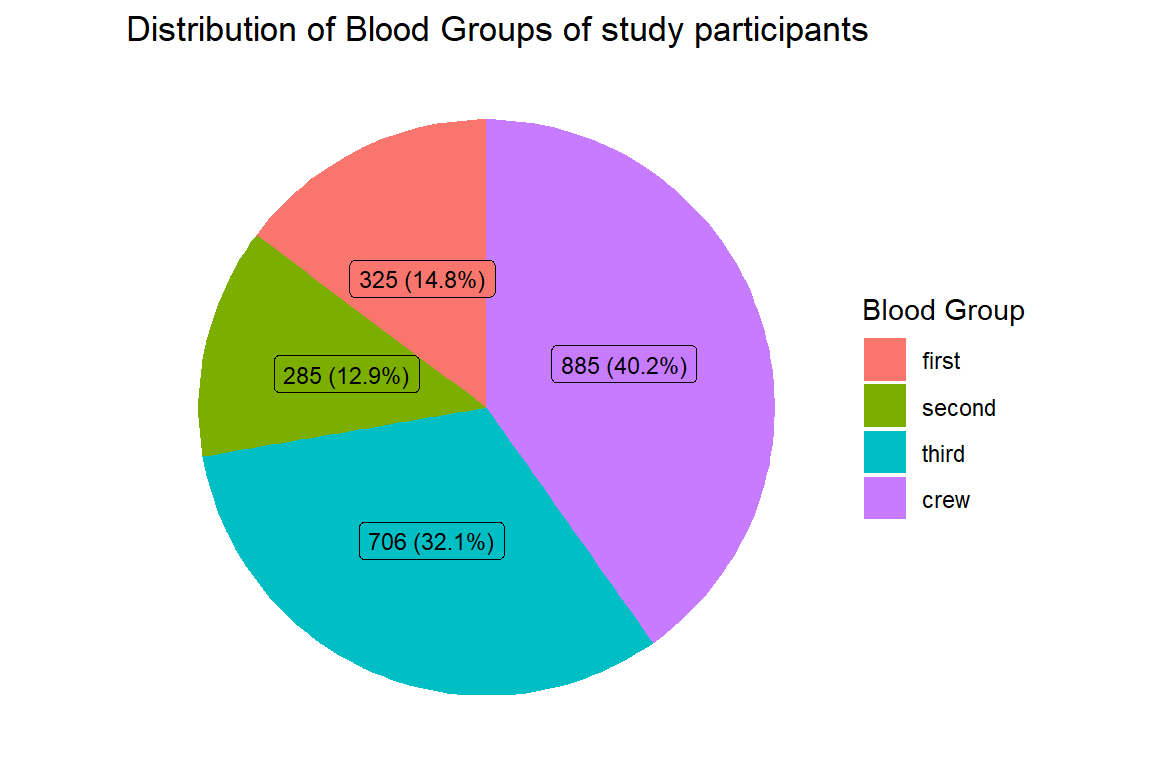
9.1.3 Two categorical Variables
9.1.3.1 Frequencies & Proportions
titanic2 %>%
tbl_cross(row = sex, col = died) %>%
bold_labels()
Died at sea | Total | ||
---|---|---|---|
No | Yes | ||
Sex of passenger | |||
female | 344 | 126 | 470 |
male | 367 | 1,364 | 1,731 |
Total | 711 | 1,490 | 2,201 |
9.1.3.2 Row percentages
titanic2 %>%
tbl_cross(row = sex, col = died, percent = "row", digits = c(0,1)) %>%
bold_labels()
Died at sea | Total | ||
---|---|---|---|
No | Yes | ||
Sex of passenger | |||
female | 344 (73.2%) | 126 (26.8%) | 470 (100.0%) |
male | 367 (21.2%) | 1,364 (78.8%) | 1,731 (100.0%) |
Total | 711 (32.3%) | 1,490 (67.7%) | 2,201 (100.0%) |
9.1.3.3 Column percentages
titanic2 %>%
tbl_cross(row = sex, col = died, percent = "col", digits = c(0,1)) %>%
bold_labels()
Died at sea | Total | ||
---|---|---|---|
No | Yes | ||
Sex of passenger | |||
female | 344 (48.4%) | 126 (8.5%) | 470 (21.4%) |
male | 367 (51.6%) | 1,364 (91.5%) | 1,731 (78.6%) |
Total | 711 (100.0%) | 1,490 (100.0%) | 2,201 (100.0%) |
9.1.3.4 Table Total Percentages
titanic2 %>%
tbl_cross(
row = sex,
col = died,
percent = c("cell"),
digits = c(0,1)) %>%
bold_labels()
Died at sea | Total | ||
---|---|---|---|
No | Yes | ||
Sex of passenger | |||
female | 344 (15.6%) | 126 (5.7%) | 470 (21.4%) |
male | 367 (16.7%) | 1,364 (62.0%) | 1,731 (78.6%) |
Total | 711 (32.3%) | 1,490 (67.7%) | 2,201 (100.0%) |